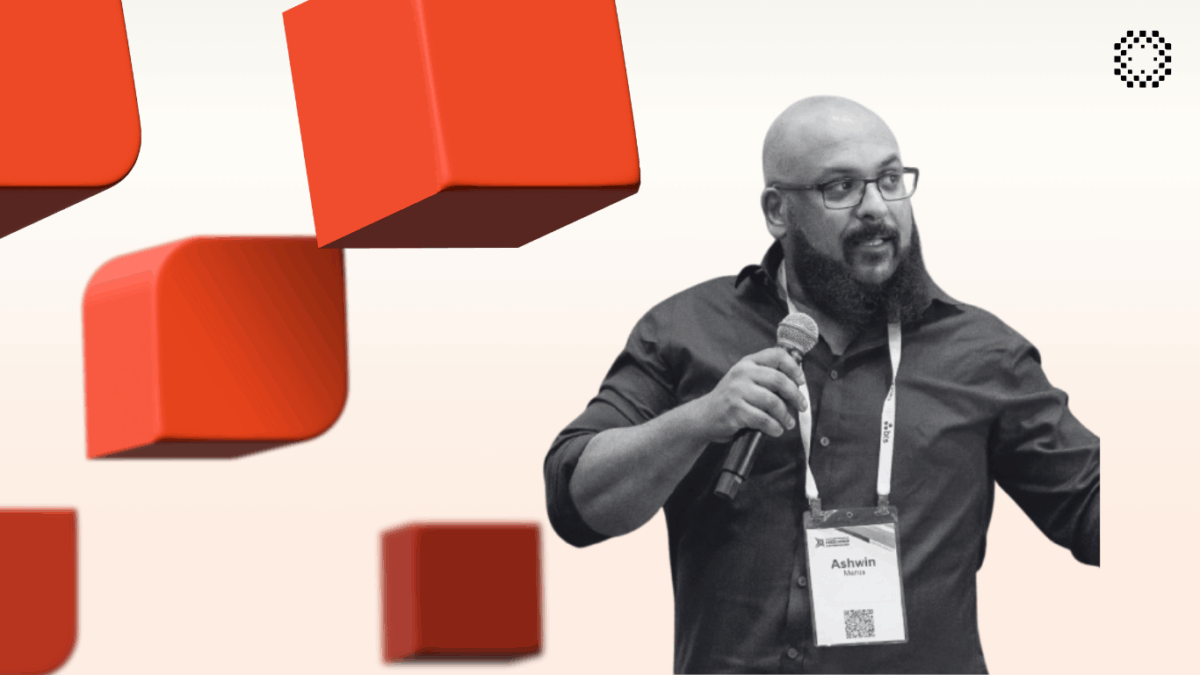
Published on 13th May 2025
Dr Ashwin Mehta on The Future of Adaptive Learning
Dr Ashwin Mehta explores the science, strategy, and future of adaptive learning in this expert Q&A on AI, behavior, and personalization.
In this interview, we speak with Dr. Ashwin Mehta—AI strategist, learning technologist, and founder of Mehtadology—about the evolving landscape of adaptive learning. From the science behind personalized pathways to its future potential, Ashwin offers deep insight into what makes adaptive a true game-changer for learning.
- To start us off, could you briefly introduce yourself and share a bit about your background and expertise in AI and learning technologies?
I’m Dr. Ashwin Mehta, the founder and CEO of Mehtadology, an AI and technology consulting firm, with a strong focus on human capital and learning. For around 14 years, I’ve worked in the learning and digital learning space, starting with roles that involved e-learning, LMS platforms, and related technologies.
Along the way, I completed an MBA and a PhD in technology adoption—specifically looking at how people interact with technology and how we can model those behaviors. I used structural equation modeling to explore these dynamics, and I also experimented with machine learning and neural networks to better understand and represent behavioral data.
I went on to work at a Big Four consultancy and later led learning tech strategy at a pharmaceutical company. Throughout, I’ve observed that the typical learning paradigm centers on three components: content, infrastructure (platforms), and data. Usually, the platforms are LMSs or LXP-type systems, and the content is largely static—offering the same material to every learner. That’s still the norm for many large organizations across industries today.
Now, through my own company, I work across a range of technology-driven projects, and I also serve as Chief AI Strategist for the Learning and Performance Institute (LPI).
- In your view, what truly defines adaptive learning? What makes it different from traditional e-learning or even personalized learning systems?
Adaptive learning is best understood as a system that adapts to learner behavior. It’s not just about content—it’s about how the system responds to what a learner does, knows, or needs in real time.
Most of the market tends to focus on content: breaking it into chunks or micro-objects to serve learners what they don’t know or need to reinforce. But to do that effectively, we need meaningful data—on what learners know, how they behave, and how they approach challenges or problem-solving.
But that’s not the full extent of adaptive in corporate learning. We can think of adaptivity across multiple layers. At the most granular level, you might have a single object that adapts to learner data. Then zooming out, you could also be thinking about an e-learning courses or modules—essentially a group of objects like videos or text—which as a whole, forms a content module, which is packaged up and put into a system. So, we can have adaptive objects and adaptive content more broadly within a course. Beyond that, we can think about how multiple modules are strung together in a journey or pathway, to think about adaptive pathways as well.
So, when we talk about “adaptive” learning we could be talking about any level of granularity in the system we have.
This layered adaptivity moves us away from a one-size-fits-all approach. Take the example of learning to drive a manual car: One learner might know exactly how to perform an emergency stop correctly the first time, while another might experiment with different pedal combinations before figuring it out. In a corporate context, those are two very different learners—one might know what they’re doing and need no further support, while the other could require additional support, especially if safety is involved.
By capturing behavioral data within the system, we can distinguish between those two learners and adapt the next step on what the learner needs accordingly. That relies on having good tagging, well-structured content, and meaningful insights from user data. When done well, adaptive learning allows us to be very tactical in how we support our learners, delivering targeted, personalized support that’s almost like a Goldilocks approach – “just right” for everyone: not too much and not too little.
- What is the science behind how adaptive learning works? How do AI, behavioral data, and metacognition come together to power these systems?
At the heart of adaptive learning is learning science—and specifically, how we differentiate between cognitive, motivational, and behavioral outcomes. But in adaptive systems, there’s an additional layer we consider: metacognition.
Metacognition is essentially “thinking about thinking.” Just like metadata describes data, metacognition reflects how learners think about their own learning processes. It’s an abstract layer: cognition is thinking, while metacognition is how learners reflect on and regulate their thinking. For example, someone might know that they learn best when they digest, reflect, play, and practice—this awareness enables better learning strategies.
Adaptive learning systems aim to capture signals of these behaviors through data: how much time a learner spends in the system, how they revisit content, how they engage with digital artifacts. From this behavioral data, we can start to infer not just what the learner knows, but how they’re thinking and engaging with the material.
While these systems are primarily about delivering the right information, there’s a growing frontier around adaptive simulations—where the experience itself evolves in response to user behavior. Video games already do this: enemies adapt based on the player’s data – their skill, progress, or equipment. Learning environments aren’t quite there yet, but the same principle can apply as the tech matures—especially in simulation-based training. The science is evolving fast, and we’re getting closer to being able to create truly responsive learning environments powered by rich behavioral and cognitive data.
- What do you think organizations find challenging when shifting from traditional linear based learning to adaptive?
Implementing adaptive learning can require a shift in thinking—especially when moving from static systems to something more dynamic. There are typically two stumbling blocks.
Firstly, it requires a shift away from the traditional mindset of “I’m going to tell the learner this, they’re going to click through it, and we’ll validate that they clicked the button.” Adaptive learning, by contrast, is based much more on cognition and metacognition, and that gives you a very different kind of data.
It also requires you to think differently about what your interventions actually are. You’re no longer just disseminating information—you’re focusing on outcomes. In environments where learning has historically focused on compliance or risk mitigation, it can be difficult to suddenly start asking: “Why do we want people to know these things? What does it mean if someone spends more or less time on a topic? How do we identify and act on trends? What does this tell us about how to reshape our learning strategy?” These are big questions and often require a different way of thinking about learning design and impact.
The second challenge is operational. It’s not just a case of lifting static content into a new system. Adaptive learning often benefits from a more strategic approach—one that starts with a clear objective, such as addressing a specific behavior or solving a business problem rooted in human factors. That starting point makes it much easier to design a learning experience that is truly adaptive, rather than trying to retrofit adaptivity into something that wasn’t built for it.
So, there are two distinct but connected challenges: the strategic mindset shift, and the operational delivery.
- As adaptive learning continues to evolve, what emerging technologies or innovations do you believe will shape its next chapter?
As adaptive learning evolves, we’re seeing a shift from platforms that just chunk up existing content to ones that embed personal, contextual, conversational agents. These systems can draw on a company’s data—whether that’s internal policies, procedures, or even manufacturing manuals—and provide responses that are tailored to the individual and the situation.
This opens up two interesting dimensions of context. First, how do the responses change dependent on a person’s context—their geography, role, skills, language—everything that makes up the learner’s profile. Second, there’s the environmental context—the job, the task, the activity, the history of the data, and current best practices. With newer generative AI systems, especially those that companies may need to build themselves, we’re increasingly capable of delivering highly personalized, accurate responses in real time.
That said, it’s not easy. You need the right expertise and protections against issues like accuracy and hallucination. But the potential for highly sophisticated adaptive systems is very real. This could fundamentally shift the market. You’ll see some systems relying on legacy data, some generating new data, and others pushing into new territory with truly responsive conversations.
The key challenge for the learning industry is to somehow move beyond SCORM and the LMS checkbox mentality. If we have nuanced, contextualized, and conversational systems, we need better ways of understanding what the data tells us—about behavior, cognition, and metacognition.
Interested in making adaptive learning a reality in your organization? Talk to our team to discover how Obrizum’s true adaptive platform can help you deliver measurable impact at scale.